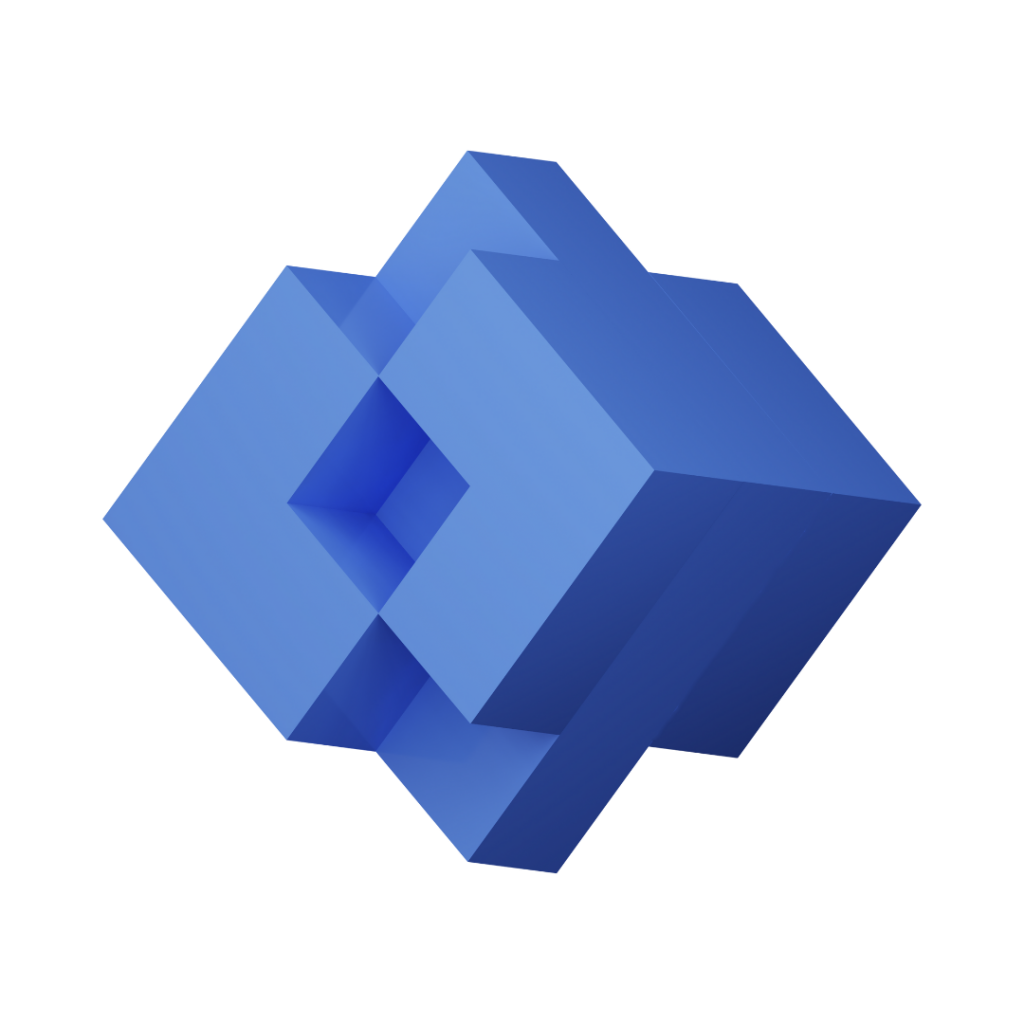
THE FOUNDATION OF INTELLIGENT DECISION-MAKING
Data Readiness
Enterprise AI and data-driven decision-making rely on high-quality, structured, and accessible data. Without a strong data foundation, AI models and analytics tools produce unreliable insights, leading to inefficiencies, misinformed strategies, and operational risks. Data-readiness ensures that organizations can fully leverage their data assets by eliminating silos, ensuring accuracy, and maintaining relevance across all functions. A well-structured data environment is the key to unlocking trustworthy, scalable, and actionable intelligence.
With a strong data foundation,
AI models and analytics tools produce reliable insights.
Ensuring Clean and Accurate Data
AI and analytics tools depend on clean, consistent, and validated data to generate meaningful insights. Poor data quality—such as inconsistencies, missing values, or outdated records—can significantly impact decision-making, leading to incorrect forecasts or flawed automation. Implementing data validation processes, automated cleansing techniques, and governance policies ensures that data remains accurate and reliable, reducing the risk of AI-driven errors.
Eliminating Redundancies
and Siloed Data
Many organizations struggle with duplicate, fragmented, or redundant data, which creates inefficiencies and distorts analytical outputs. Redundant data increases storage costs, complicates data management, and introduces inconsistencies between systems. A non-siloed, integrated approach to data management eliminates redundancies and enables organizations to access a single, unified version of the truth. This not only enhances efficiency but also improves collaboration across departments and business units.
Structuring Data for
Relevance and Context
Not all data is valuable for decision-making. AI models require contextually relevant, domain-specific data to deliver precise and meaningful insights. Organizations must prioritize data classification, metadata tagging, and contextual alignment to ensure that AI systems are processing the most relevant information. A structured approach to data ensures that businesses extract actionable intelligence that aligns with their strategic objectives.
The Role of Information Modeling
in Data-Readiness
Effective information modeling is essential for creating a structured data environment where AI and analytics can thrive. By defining data relationships, hierarchies, and metadata structures, organizations ensure that their data is both interoperable and interpretable by AI systems. Information modeling helps establish standardized taxonomies, governance frameworks, and logical data flows, reducing complexity and ensuring consistency across enterprise applications.
Prototyping for
Continuous Improvement
Before fully deploying AI-driven solutions, organizations must prototype and test data pipelines to ensure their models work as intended. Prototyping allows businesses to validate data quality, refine governance structures, and optimize AI performance before scaling implementation. This iterative approach minimizes risks, enhances adaptability, and ensures that AI-driven insights remain accurate, scalable, and aligned with business needs. By prioritizing data-readiness, organizations create a future-proof data strategy that drives innovation, efficiency, and long-term success.